Harnessing Deep Learning for Enhanced Weather Predictions
Written on
Chapter 1: The Climate Challenge
In 2019, Time magazine honored Greta Thunberg, a young climate activist, as its ‘Person of the Year.’ With her youthful energy and relentless dedication to environmental preservation, Greta has been vocal about the urgent threat posed by climate change—a crisis often described as existential in nature, largely driven by human actions.
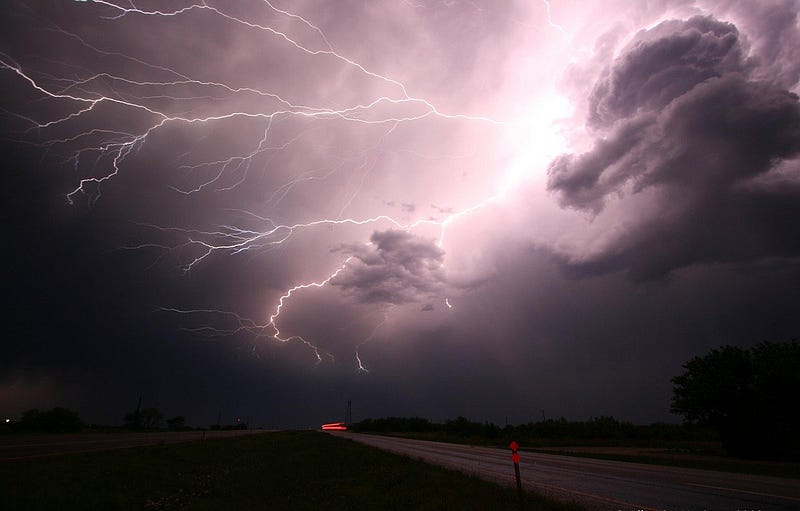
To grasp the consequences of such a significant global issue, we require extensive scientific data, advanced visualization tools, and reliable predictive models. Consequently, weather forecasting and climate modeling are critical in humanity’s response to climate change. However, these tasks are far from simple. Despite advancements in Big Data analytics and scientific simulations, solving these Earth-scale problems remains a challenge for current technology.
Can we leverage artificial intelligence, particularly deep neural networks, to assist in this endeavor?
Section 1.1: The Role of Deep Learning
Deep learning significantly accelerates this complex undertaking.
The Weather Research and Forecasting (WRF) Model
In the U.S., the primary framework for weather predictions is a sophisticated mesoscale model known as the Weather Research and Forecasting (WRF) model. This model caters to a diverse array of meteorological applications, spanning from local to regional scales. As environmental scientist Jiali Wang from the U.S. Department of Energy’s Argonne National Laboratory aptly states, “It captures everything visible outside your window—from clouds to solar radiation, snow, and even how skyscrapers influence wind patterns.”
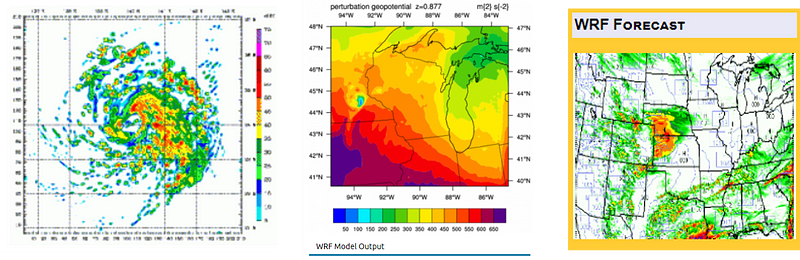
The WRF model was developed in the late 1990s through collaboration among various governmental agencies and universities, including the National Center for Atmospheric Research (NCAR) and the National Oceanic and Atmospheric Administration (NOAA). Today, it boasts a vast global user base of over 30,000 registered users across more than 150 countries, supported by workshops and tutorials throughout the year. It serves not only as a research tool but also for real-time forecasting, equipped with two dynamical cores and a data assimilation system.
Section 1.3: The Data-Driven Future
DNNs thrive on vast amounts of data, and fortunately, the Argonne Leadership Computing Facility (ALCF) offers an abundance of it. As part of the Department of Energy's user facilities, ALCF utilizes powerful supercomputers to generate meteorological data from intricate simulations. Coupled with high-performance computing resources from the National Energy Research Scientific Computing Center, researchers have access to nearly a petabyte of data, representing 300 years of North American weather history for training their DNNs.
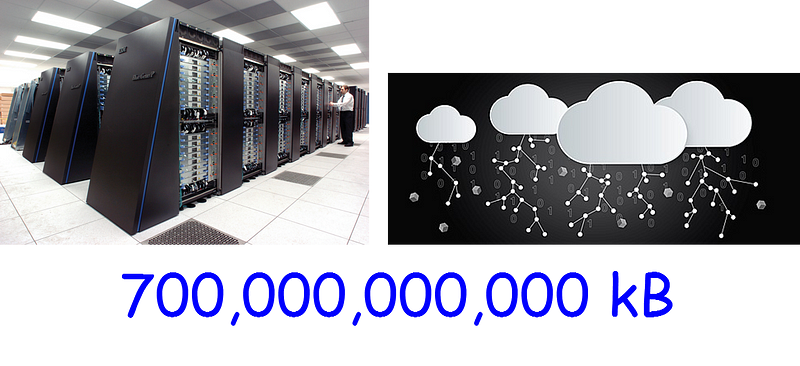
Summary
The reality of climate change stands as one of the most formidable challenges faced by humanity. Yet, revolutionary technologies such as artificial intelligence, alongside Cloud and Big Data analytics, present tremendous opportunities for innovation and solutions. Can we harness these advancements to address the climate crisis? This article highlights a significant scientific initiative aimed at achieving that goal, among many others.
The second video discusses the integration of AI and machine learning in weather and climate modeling, shedding light on their transformative potential.
If you have any questions or ideas to share, please contact the author at tirthajyoti[AT]gmail.com. You can also explore the author's GitHub repositories for code and resources related to machine learning and data science. If you share a passion for AI, machine learning, or data science, feel free to connect with the author on LinkedIn or follow on Twitter.
Tirthajyoti Sarkar - Senior Principal Engineer - Semiconductor, AI, Machine Learning - ON…
www.linkedin.com